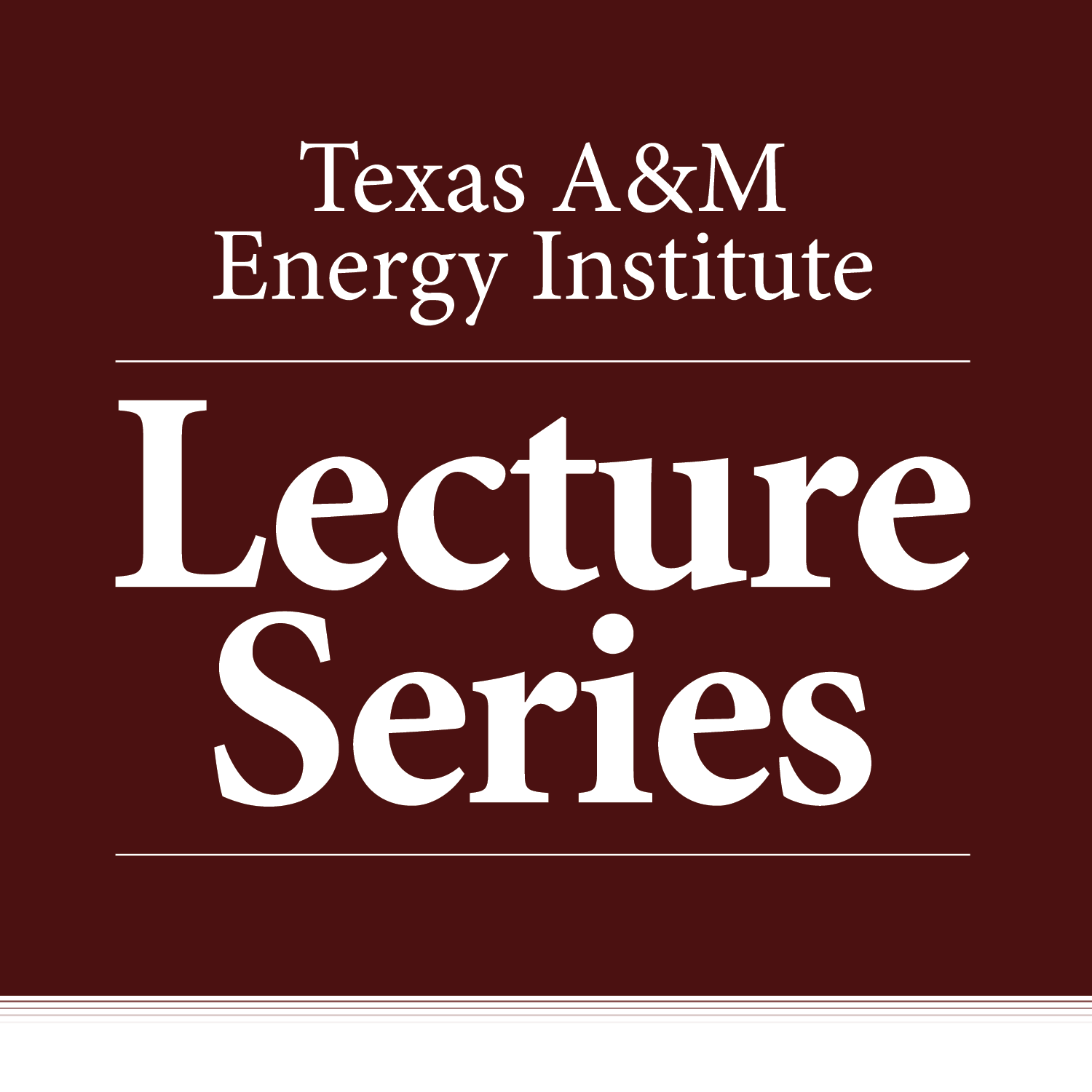
“Recent Progress in Machine Learning and Implications for the Process and Energy Systems Engineering Fields”
The next presentation in the Texas A&M Energy Institute Lecture Series, featuring Dr. Jay H. Lee, a professor in the Department of Chemical and Biomolecular Engineering and Director of Aramco-KAIST CO2 Management Center at the Korea Advanced Institute of Science and Technology (KAIST), will be held on Tuesday, September 19, 2017 from 1:30 p.m. – 3:00 p.m. in the Frederick E. Giesecke Engineering Research Building (GERB) Third Floor Conference Room. The topic will be “Recent Progress in Machine Learning and Implications for the Process and Energy Systems Engineering Fields.”
Abstract
Machine learning has recently come into limelight and its popularity is spurred by advances in deep learning, reinforcement learning, GPU-based computing, and commercial interest in big data and predictive analytics. Despite the enthusiasm, some renowned experts of the filed have expressed skepticism, which is justifiable given the previous disappointment with neural networks and other AI techniques. On the other hand, new fundamental advances like the ability to train neural networks with a large number of layers for hierarchical feature learning can bring significant new opportunities. In this presentation, I will critically examine the main motivation behind and advances in deep learning. I will also discuss the recent advances in another branch of machine learning called ‘reinforcement learning’ and its relationship with dynamic programming and self-optimizing simulation. Implications of these advances for the fields of process and energy systems engineering are discussed and the problem of integrated planning and operation of a hybrid renewable energy network is used to illustrate the potential of the approach.
The seminar will also include a brief introduction of KAIST and activities at Aramco-KAIST CO2 Management Center and Chemical and Biomolecular Engineering Department.
Biography
Jay H. Lee obtained his B.S. degree in Chemical Engineering from the University of Washington, Seattle, in 1986, and his Ph.D. in Chemical Engineering from the California Institute of Technology, Pasadena, in 1991.
From 1991 to 1998, he was with the Department of Chemical Engineering at Auburn University, AL, as an Assistant Professor and an Associate Professor. From 1998-2000, he was with School of Chemical Engineering at Purdue University, West Lafayette, and then with the School of Chemical Engineering at Georgia Institute of Technology, Atlanta from 2000-2010.
Since 2010, he has been with the Chemical and Biomolecular Engineering Department at the Korea Advanced Institute of Science and Technology (KAIST), where he was the department head from 2010-2015. He is currently a Professor, Associate Vice President of International Office, and Director of Saudi Aramco-KAIST CO2 Management Center.
He has held visiting appointments at E. I. Du Pont de Numours, Wilmington, in 1994 and at Seoul National University, Seoul, Korea, in 1997. He was a recipient of the National Science Foundation’s Young Investigator Award in 1993 and was elected as an IEEE Fellow and an IFAC (International Federation of Automatic Control) Fellow in 2011 and AIChE Fellow in 2013. He was also the recipient of the 2013 Computing in Chemical Engineering Award given by the AIChE’s CAST Division and the 2016 Roger Sargent Lecturer at Imperial College, UK. He is currently an Editor of Computers and Chemical Engineering and also the chair of IFAC Technical Committee on Chemical Process Control. He published over 170 manuscripts in SCI journals with more than 12000 Google Scholar citations (H-index of 48). His research interests are in the areas of system identification, state estimation, robust control, model predictive control and approximate dynamic programming with applications to energy systems, bio-refinery, and CO2 capture/conversion systems.