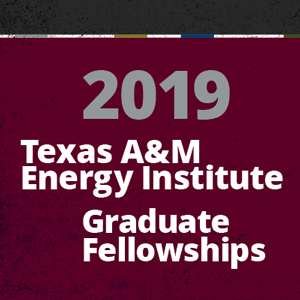
The Texas A&M Energy Institute is proud to announce the naming of the 2019-20 Energy Institute Fellows.
These fellowships aim to reward excellence in energy research, promote future research that is important to our energy future, and encourage students to pursue careers in energy.
These graduate fellowships recognize outstanding energy research work performed by Ph.D. students under the supervision of Affiliated Faculty Members of the Texas A&M Energy Institute.
Process intensification refers to the development of chemical processing techniques and equipment that lead to substantially smaller, cleaner, safer, and more energy-efficient processes compared to their traditional counterparts. Often times, intensification alternatives are not known beforehand and identification of such novel solutions during the conceptual design stage, where the initial layout of the chemical plant is decided, necessitates systematic design methodologies. To this end, we developed an optimization-based method for the discovery of new chemical process designs. This method relies on an original representation of chemical process operations using building blocks. These blocks represent the fundamental physicochemical phenomena, tasks and unit operations that constitute most of the processes used in the chemical process industry (CPI). Various combinations of building blocks can yield a plethora of new equipment and flowsheets. A mixed-integer nonlinear programming (MINLP) optimization model is used to describe the systematic selection of optimal block combinations towards generating an intensified process system. The MINLP model incorporates mass and energy conservations, and descriptions of reaction, separation and material selection. With this generic optimization model, building block-based design methodology provides an automated approach for generation and screening of novel intensified process alternatives.
Turbulent flows are ubiquitous in nature and engineering systems and are critical to the efficient production and consumption of energy in conventional and future energy systems. Turbulence has first-order effects on critical processes, such as mixing of fuel and oxidizers, drag over cars and airplanes, and power generation from wind energy. Yet, turbulence is a notoriously difficult problem, especially at realistic conditions, due to a wide range of interacting spatial and temporal scales. Fundamental understanding of these interactions is currently lacking and is critical to developing predictive capabilities for turbulent systems. We use massively parallel supercomputers for direct numerical simulations (DNS) to solve the exact equations of fluid motion across all scales, without any modeling, and with a resolution an order of magnitude larger than traditional DNS. This provides a new window into the finest scales of turbulence, largely unstudied yet, and their interactions. We are also developing a theoretical formalism to predict the statistical behavior of turbulence at realistic flow conditions by understanding the behavior of the finest scales at smaller parameter ranges. These parameter ranges and resolutions are computationally accessible on the largest supercomputers today, unlike flows at realistic conditions. In doing so, we aim to further our understanding of dynamics within different scales of turbulent flows and advance the theoretical development of exact theories as well as low-fidelity models of turbulence for engineering design and computational predictive capabilities.
Algae-based biofuels have been regarded as an ultimate solution for renewable energy, considering the potentially high productivity, the efficient energy conversion from sunlight, the capacity to capture and utilize CO2 at high conversion rates, and the replacement of fossil fuels with limited land usage. Despite extensive efforts, the scale-up and commercialization of algal biofuel are still hindered by several fundamental challenges, including low light penetration, costly dewatering and the requirement to extract algal oil. In my graduate research, I designed an auto-flocculation-based continuous hydrocarbon and carbohydrate co-production platform to address these challenges. In the platform, the cell surface structure of cyanobacteria was modified to enable auto-flocculation, which can be applied to reduce costs for biomass harvesting and transform cyanobacterial biofuel from batch production to continuous production. Meanwhile, a limonene synthase was introduced into the strain to catalyze limonene biosynthesis, enabling continuous limonene and glycogen (in biomass) co-production. Since the cell density of cyanobacteria can be tightly controlled in continuous production, light penetration efficiency could be significantly improved, leading to dramatic increases in both limonene and glycogen productivities. Next, I am focusing on: 1) to continue to optimize and scale up the Continuous Ultra-high-yield Co-production of Hydrocarbon and Carbohydrate to achieve long-term sustainable production; and 2) to define the biochemical and metabolic limits for terpene production and further improve terpene and glycogen productivities by systemically optimizing carbon and energy partition from photosynthesis to hydrocarbon and carbohydrate biosynthesis.
The adverse effects of pollution on the environment continue to be a central concern among environmental sociologists and environmental justice scholars. The negative effects of environmental pollution on vulnerable populations and the effects of organizational characteristics on pollution levels have been well documented. However, less attention has been given to the joint effects of social vulnerability and organizational characteristics on pollution. Further, few studies examine the effects of these sociological variables on greenhouse gas (GHG) emissions. To fill this gap in the literature, this project examines the effects of sociological variables on GHG emissions from all-electric power plants in Harris County and surrounding counties. There are three interrelated questions addressed in this research: (1) How do organizational characteristics affect pollution levels among electric power plants? (2) How do community-level social vulnerability characteristics affect pollution levels? (3) How does the interaction between organizational and social vulnerability characteristics affect pollution levels? Geographic information systems (GIS) spatial analyses and regressions are used to test for the effects of organizational variables (e.g., organizational complexity and organizational type) and social vulnerability variables (e.g., plant location relative to low-income and majority-minority race communities, community income level, and community percent minority).
Citing energy and environmental reasons, many individuals argue that it is time to adopt renewable energy sources (RES) and transform grids into smarter grids. The deployment and integration of fluctuating renewable energy into the power grid and the eventual transformation into a smart grid will require the use of advanced technologies and management strategies. My research is focused on the development of a real-time big data analytics platform for both the current grid and for future smart grids. The project focuses on developing big data analytics, predictive analytics, forecasting analytics, cyber-physical security and privacy for accurate and efficient energy consumption forecasting. This will lead to the development and testing of a dynamic energy management system with a big data analytics platform, which will help in the optimization of energy resources and load management for both energy efficiency and demand response programs based on real-time processing. Big data analytics and machine learning will help integrate renewable energy sources with energy storage and forecast services while considering uncertainty and customer behavior. Also, mining information from big data available from smart grids makes it possible to prevent blackouts, as it enables the discovery of underlying patterns and an understanding of the highly complex structures and thousands of system variables of a power network, thus paving the way to accurately predict the unstable status of power network components in-time. Currently, I am working on the applications of load forecasting and voltage stability analysis using deep learning models. In tandem, centralized and distributed control strategies are being worked upon and a combination of the RTP program with the IBR model will be used as the most efficient model for energy demand management.